技术专栏
关于运动跟踪及行人检测数据集的介绍
DanceTrack 运动跟踪数据集
简介
DanceTrack 是一个大规模的多对象跟踪数据集。用于在遮挡、频繁交叉、同样服装和多样化身体姿态条件下对人进行跟踪。强调运动分析在多对象跟踪中的重要性。
GitHub地址:https://github.com/DanceTrack/DanceTrack
数据集下载地址:https://pan.baidu.com/s/19O3IvYNzzrcLqlODHKYUwA
提取码:awew
转为Labelme标注的物体检测数据集格式
import sys
import base64
import os
import cv2
import shutil
import glob
module_path = os.path.abspath(os.path.join('..'))
if module_path not in sys.path:
sys.path.append(module_path)
import json
from PIL import Image
Image.MAX_IMAGE_PIXELS = None
xmlpathNames_path='../train1/*/gt/gt.txt'
xmlpathNames=glob.glob(xmlpathNames_path)
print(xmlpathNames)
version = '3.16.7'
flags = {}
lineColor = [0, 255, 0, 128]
fillColor = [255, 0, 0, 128]
image_t='../images/'
os.makedirs(image_t,exist_ok=True)
for xmlpathName in xmlpathNames:
xmlpathName=xmlpathName.replace("\\","/")
dancetrack_name=xmlpathName.split("/")[-3]
dic_info = {}
with open(xmlpathName) as fs:
lines = fs.readlines()
lines = sorted(lines)
for line in lines:
line = line.replace("\n", '')
line_info = line.split(',')
frame = line_info[0]
frame_image_name = '{:0>8d}'.format(int(frame)) + ".jpg"
box = [int(line_info[2]), int(line_info[3]), int(line_info[2]) + int(line_info[4]),
int(line_info[3]) + int(line_info[5])]
if frame_image_name in dic_info:
box_list = dic_info[frame_image_name]
box_list.append(box)
dic_info[frame_image_name] = box_list
else:
box_list = [box]
dic_info[frame_image_name] = box_list
for image_name in dic_info.keys():
dic = {}
dic['version'] = version
dic['flags'] = flags
dic['shapes'] = []
img_path = "../train1/"+dancetrack_name+"/img1/" + image_name
img_new_name = dancetrack_name + "_" + image_name
img_new_path = image_t + img_new_name
try:
shutil.copy(img_path, image_t + img_new_name)
except :
continue
img = cv2.imread(img_new_path)
imageHeight, imageWidth, _ = img.shape
for data in dic_info[image_name]:
shape = {}
shape['label'] = 'person'
shape['line_color'] = None
shape['fill_color'] = None
x1 = int(data[0])
y1 = int(data[1])
x2 = int(data[2])
y2 = int(data[3])
shape['points'] = [[x1, y1], [x2, y2]]
shape['shape_type'] = 'rectangle'
shape['flags'] = {}
dic['shapes'].append(shape)
dic['lineColor'] = lineColor
dic['fillColor'] = fillColor
dic['imagePath'] = img_new_name
dic['imageData'] = base64.b64encode(
open('{}'.format(img_new_path), "rb").read()).decode('utf-8')
dic['imageHeight'] = imageHeight
dic['imageWidth'] = imageWidth
fw = open('{}json'.format(img_new_path.replace(img_new_path.split('.')[-1], "")), 'w')
json.dump(dic, fw)
fw.close()
WiderPerson行人检测数据集
简介
WiderPerson 是关于户外行人检测的基准数据集。该数据集图像场景多样,不再局限于交通场景。该数据集包含 13,382 张图像,40 万个遮挡物的标注,其中 8,000 张图像用于训练,1,000 张图像用于验证,4,382 张图像用于测试。与 CityPersons 和 WIDER FACE 数据集类似,该数据集不公布测试图像的 bounding box ground truth。该数据集包含密集的行人和各种遮挡,适合进行户外环境的行人检测评估。
官网地址:http://www.cbsr.ia.ac.cn/users/sfzhang/WiderPerson/
百度网盘:https://pan.baidu.com/s/1ulMlbw_zhNUYwdyXONLrwg
提取码:uq3u
转为Labelme标注的物体检测数据集格式
import os
import numpy as np
import scipy.io as sio
import shutil
from lxml.etree import Element, SubElement, tostring
from xml.dom.minidom import parseString
import cv2
import base64
import json
if __name__ == '__main__':
# < class_label =1: pedestrians > 行人
# < class_label =2: riders > 骑车的
# < class_label =3: partially-visible persons > 遮挡的部分行人
# < class_label =4: ignore regions > 一些假人,比如图画上的人
# < class_label =5: crowd > 拥挤人群,直接大框覆盖了
version = '3.16.7'
flags = {}
lineColor = [0, 255, 0, 128]
fillColor = [255, 0, 0, 128]
classes = {'1': 'person',
'2': 'person',
'3': 'person',
'4': 'person',
'5': 'person',
# 不需要哪个类的话直接删去
} # 这里如果自己只要人,可以把1-5全标记为people,也可以根据自己场景需要筛选
VOCRoot = './images/' # 生成的voc2007的位置
os.makedirs(VOCRoot,exist_ok=True)
widerDir = './WiderPerson' # widerperson文件夹所在的路径
wider_path = './WiderPerson/train.txt' # widerperson文件夹所中训练集+验证集txt标签所在位置
with open(wider_path, 'r') as f:
imgIds = [x for x in f.read().splitlines()]
for imgId in imgIds:
objCount = 0 # 一个标志位,用来判断该img是否包含我们需要的标注
filename = imgId + '.jpg'
img_path = './WiderPerson/images/' + filename
file_new_name='wider_'+filename
print('Img :%s' % img_path)
img = cv2.imread(img_path)
width = img.shape[1] # 获取图片尺寸
height = img.shape[0] # 获取图片尺寸 360
dic = {}
dic['version'] = version
dic['flags'] = flags
dic['shapes'] = []
label_path = img_path.replace('images', 'Annotations') + '.txt'
with open(label_path) as file:
line = file.readline()
count = int(line.split('\n')[0]) # 里面行人个数
lines = file.readlines()
for line in lines:
cls_id = line.split(' ')[0]
if cls_id not in classes:
print(cls_id)
continue
shape = {}
shape['label'] = cls_name = classes[cls_id]
shape['line_color'] = None
shape['fill_color'] = None
x1 = int(line.split(' ')[1]) + 1
y1 = int(line.split(' ')[2]) + 1
x2 = int(line.split(' ')[3]) + 1
y2 = int(line.split(' ')[4].split('\n')[0]) + 1
shape['points'] = [[x1, y1], [x2, y2]]
shape['shape_type'] = 'rectangle'
shape['flags'] = {}
dic['shapes'].append(shape)
dic['lineColor'] = lineColor
dic['fillColor'] = fillColor
dic['imagePath'] = filename
dic['imageData'] = base64.b64encode(
open('{}'.format(img_path), "rb").read()).decode('utf-8')
dic['imageHeight'] = height
dic['imageWidth'] = width
suffix=(VOCRoot + file_new_name).split('.')[-1]
fw = open('{}'.format((VOCRoot + file_new_name).replace(suffix,"json")), 'w')
json.dump(dic, fw)
fw.close()
shutil.copy(img_path, VOCRoot + file_new_name)
声明:本文内容由易百纳平台入驻作者撰写,文章观点仅代表作者本人,不代表易百纳立场。如有内容侵权或者其他问题,请联系本站进行删除。
红包
点赞
收藏
评论
打赏
- 分享
- 举报
评论
0个
手气红包
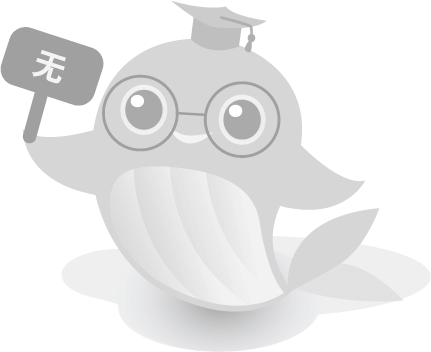
相关专栏
-
浏览量:4632次2021-02-04 16:47:25
-
浏览量:65次2024-03-05 16:55:32
-
浏览量:486次2024-02-05 10:11:42
-
浏览量:308次2023-09-08 14:00:44
-
浏览量:1695次2023-02-17 11:37:20
-
浏览量:1686次2018-01-17 12:06:58
-
浏览量:9702次2021-04-27 00:28:09
-
浏览量:384次2023-11-18 14:30:59
-
浏览量:3069次2019-11-13 09:07:39
-
浏览量:629次2023-01-12 17:13:47
-
浏览量:1305次2023-04-14 10:12:00
-
浏览量:4915次2021-05-02 18:00:46
-
浏览量:5134次2021-02-18 16:03:22
-
浏览量:106次2024-03-14 18:20:47
-
浏览量:3935次2021-01-28 17:24:58
-
浏览量:288次2023-09-01 11:37:24
-
浏览量:1471次2023-02-14 20:27:20
-
浏览量:997次2023-11-25 17:47:33
-
浏览量:551次2023-10-09 18:13:57
置顶时间设置
结束时间
删除原因
-
广告/SPAM
-
恶意灌水
-
违规内容
-
文不对题
-
重复发帖
打赏作者
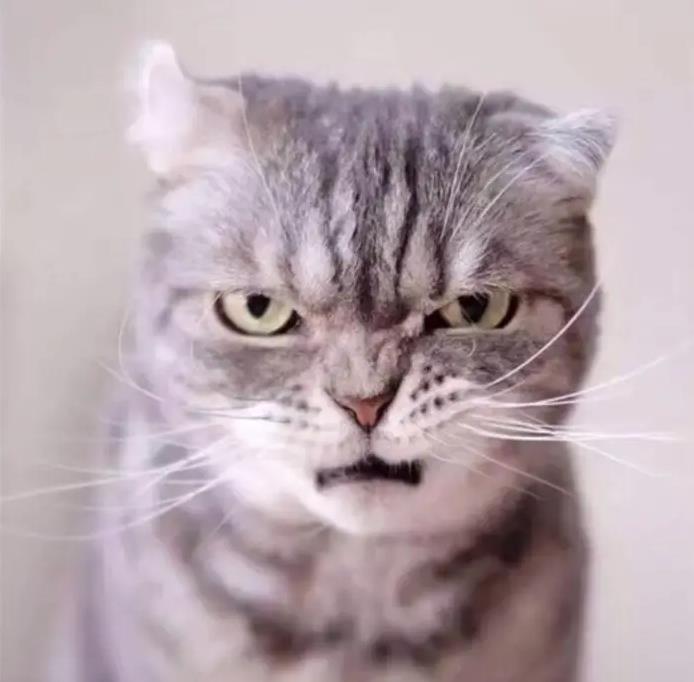
风清扬
您的支持将鼓励我继续创作!
打赏金额:
¥1

¥5

¥10

¥50

¥100

支付方式:

举报反馈
举报类型
- 内容涉黄/赌/毒
- 内容侵权/抄袭
- 政治相关
- 涉嫌广告
- 侮辱谩骂
- 其他
详细说明
审核成功
发布时间设置
发布时间:
请选择发布时间设置
是否关联周任务-专栏模块
审核失败
失败原因
请选择失败原因
备注
请输入备注