【深度学习】keras框架使用预训练模型进行Finetune的应用
文章目录
1 概述
2 Keras
3 VGG16 (VGG16)
4 MobileNetV2 (MobileNetV2)
5 盗版ResNetV2 (InceptionResNetV2)
6 盗梦空间V3 (InceptionV3)
7 使用预训练的模型进行图像分类fine-tuning
1 概述
(1)预训练权重的作用:
预测
特征提取
微调
(2)Finetune过程:
构建图结构,截取目标张量,添加新层
加载目标张量权重
训练新层
全局微调
2 Keras
keras的finetune方法很简单。Keras的applications模块中就提供了带有预训练权重的深度学习模型。该模块会根据参数设置,自动检查本地的~/.keras/models/目录下是否含有所需要的权重,没有时会自动下载,在notebook上下载会占用notebook线程资源,不太方便,因此也可以手动wget。
#-----------------------------------构建模型------------------------------------
from keras.applications.mobilenet import MobileNet
from keras.layers import Input, Reshape, AvgPool2D,\
Dropout, Conv2D, Softmax, BatchNormalization, Activation
from keras import Model
## 加载预训练权重,输入大小可以设定,include_top表示是否包括顶层的全连接层
base_model = MobileNet(input_shape=(128,128,3), include_top=False)
## 添加新层,get_layer方法可以根据层名返回该层,output用于返回该层的输出张量tensor
with tf.name_scope("output"):
x = base_model.get_layer("conv_dw_6_relu").output
x = Conv2D(256,kernel_size=(3,3))(x)
x = Activation("relu")(x)
x = AvgPool2D(pool_size=(5,5))(x)
x = Dropout(rate=0.5)(x)
x = Conv2D(10,kernel_size=(1,1),)(x)
predictions = Reshape((10,))(x)
## finetune模型
model = Model(inputs=base_model.input, outputs=predictions)
#-------------------------------------训练新层-----------------------------------
## 冻结原始层位,在编译后生效
for layer in base_model.layers:
layer.trainable = False
## 设定优化方法,并编译
sgd = keras.optimizers.SGD(lr=0.01)
model.compile(optimizer=sgd,loss="categorical_crossentropy")
‘’‘可选:记录模型训练过程、数据写入tensorboard
callback = [keras.callbacks.ModelCheckpoint(filepath="./vibration_keras/checkpoints",monitor="val_loss"),
keras.callbacks.TensorBoard(log_dir="./vibration_keras/logs",histogram_freq=1,write_grads=True)]
’‘’
## 训练
model.fit(...)
#--------------------------------------全局微调-----------------------------------
## 设定各层是否用于训练,编译后生效
for layer in model.layers[:80]:
layer.trainable = False
for layer in model.layers[80:]:
layer.trainable = True
# 设定优化方法,并编译
sgd = keras.optimizer.SGD(lr=0.0001)
model.compile(optimizer=sgd, loss="categorical_crossentropy")
## 训练
model.fit(...)
3 VGG16 (VGG16)
VGG16 is another pre-trained model. It is also trained using ImageNet. The syntax to load the model is as follows −
VGG16是另一种预训练模型。 它还使用ImageNet进行了培训。 加载模型的语法如下-
keras.applications.vgg16.VGG16(
include_top = True,
weights = 'imagenet',
input_tensor = None,
input_shape = None,
pooling = None,
classes = 1000
)
The default input size for this model is 224x224.
该模型的默认输入大小为224x224。
4 MobileNetV2 (MobileNetV2)
MobileNetV2 is another pre-trained model. It is also trained uing ImageNet.
MobileNetV2是另一种预先训练的模型。 它还使用ImageNet进行了培训。
The syntax to load the model is as follows −
加载模型的语法如下-
keras.applications.mobilenet_v2.MobileNetV2 (
input_shape = None,
alpha = 1.0,
include_top = True,
weights = 'imagenet',
input_tensor = None,
pooling = None,
classes = 1000
)
Here,
这里,
alpha controls the width of the network. If the value is below 1, decreases the number of filters in each layer. If the value is above 1, increases the number of filters in each layer. If alpha = 1, default number of filters from the paper are used at each layer.
alpha控制网络的宽度。 如果该值小于1,则减少每层中的过滤器数量。 如果该值大于1,则增加每层中的过滤器数量。 如果alpha = 1,则在每一层使用纸张的默认过滤器数量。
The default input size for this model is 224x224.
该模型的默认输入大小为224x224 。
5 盗版ResNetV2 (InceptionResNetV2)
InceptionResNetV2 is another pre-trained model. It is also trained using ImageNet. The syntax to load the model is as follows −
InceptionResNetV2是另一个预先训练的模型。 它还使用ImageNet进行了培训。 加载模型的语法如下-
keras.applications.inception_resnet_v2.InceptionResNetV2 (
include_top = True,
weights = 'imagenet',
input_tensor = None,
input_shape = None,
pooling = None,
classes = 1000)
This model and can be built both with ‘channels_first’ data format (channels, height, width) or ‘channels_last’ data format (height, width, channels).
此模型可以使用“ channels_first”数据格式(通道,高度,宽度)或“ channels_last”数据格式(高度,宽度,通道)构建。
The default input size for this model is 299x299.
该模型的默认输入大小为299x299 。
6 盗梦空间V3 (InceptionV3)
InceptionV3 is another pre-trained model. It is also trained uing ImageNet. The syntax to load the model is as follows −
InceptionV3是另一个预先训练的模型。 它还使用ImageNet进行了培训。 加载模型的语法如下-
keras.applications.inception_v3.InceptionV3 (
include_top = True,
weights = 'imagenet',
input_tensor = None,
input_shape = None,
pooling = None,
classes = 1000
)
Here,
这里,
The default input size for this model is 299x299.
该模型的默认输入大小为299x299 。
Keras是非常简单,可扩展且易于实现的神经网络API,可用于构建具有高级抽象的深度学习应用程序。 Keras是深层倾斜模型的最佳选择。
7 使用预训练的模型进行图像分类fine-tuning
这里我实验用的数据集有三个类别,每个类别200张图片用于train,50张图片用于validation。
使用VGG16在imagenet上的预训练模型,keras里都有提供,本地没有会自动从网上下载。
冻结所有层
这里冻结了除top层的所有层。
vgg_conv = VGG16(weights='imagenet', include_top=False, input_shape=(image_size, image_size, 3))
for layer in vgg_conv.layers[:]:
layer.trainable = False
for layer in vgg_conv.layers:
print(layer, layer.trainable)
创建模型,添加新层。
model = models.Sequential()
model.add(vgg_conv)
model.add(layers.Flatten())
model.add(layers.Dense(1024, activation='relu'))
model.add(layers.Dropout(0.5))
model.add(layers.Dense(3, activation='softmax'))
model.summary()
冻结除最后三个卷积层的所有层
就是将freeze的部分改成:
vgg_conv = VGG16(weights='imagenet', include_top=False, input_shape=(image_size, image_size, 3))
for layer in vgg_conv.layers[:-4]:
layer.trainable = False
for layer in vgg_conv.layers:
print(layer, layer.trainable)
现在的学习曲线:
- 分享
- 举报
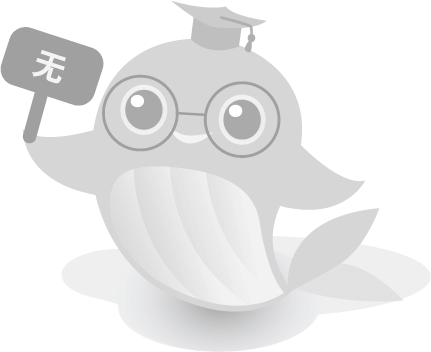
-
浏览量:7464次2021-07-13 10:59:24
-
浏览量:5146次2021-05-17 16:52:58
-
2023-07-05 10:13:58
-
浏览量:393次2023-07-14 14:21:54
-
浏览量:823次2023-03-29 10:55:15
-
浏览量:6247次2021-07-12 11:03:00
-
浏览量:4700次2021-04-21 17:06:33
-
浏览量:775次2023-06-08 10:35:09
-
浏览量:5233次2021-07-15 10:44:33
-
浏览量:171次2023-08-23 09:24:30
-
浏览量:216次2023-08-03 15:58:40
-
浏览量:303次2023-08-22 15:12:16
-
浏览量:123次2023-08-23 08:46:26
-
浏览量:5404次2021-07-30 10:33:41
-
浏览量:189次2023-07-30 17:57:28
-
浏览量:4144次2021-04-09 16:28:04
-
浏览量:285次2023-08-02 20:35:16
-
浏览量:1455次2022-05-24 17:40:33
-
浏览量:353次2023-07-24 11:00:24
-
广告/SPAM
-
恶意灌水
-
违规内容
-
文不对题
-
重复发帖
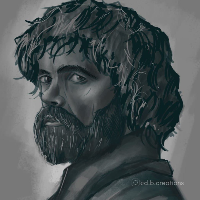
这把我C






举报类型
- 内容涉黄/赌/毒
- 内容侵权/抄袭
- 政治相关
- 涉嫌广告
- 侮辱谩骂
- 其他
详细说明