利用yolov5实现口罩佩戴检测算法并转换瑞芯微模型
文章目录
一、参考文献
利用yolov5实现口罩佩戴检测算法(非常详细)
目标检测—数据集格式转化及训练集和验证集划分
二、下载数据集
在这附上博主用的口罩数据集链接:https://pan.baidu.com/s/1Gud8jemSCdjG00TYA74WpQ
提取码:sv74
下载之后是mask.zip,解压之后是有两个文件夹images和labels,images是图片这里大概8000张图片,这里的lables已经是txt(yolo的训练标签就是txt),而一般的标签都是xml格式。标签:0:no-mask,1:mask
三、转换数据集
这呢,推荐大家去看炮哥的这篇博客,目标检测—数据集格式转化及训练集和验证集划分
这里因为博主用的数据集因为标签已经是txt格式了,但我先将txt转xml格式,再用代码直接将xml格式转为yolo(txt)格式并划分训练集和测试集。(这里不直接用txt的格式直接划分,炮哥是这样解释的,txt划分后放入训练会出错)
本例子中我的做法将区别于他们所有人的做法,我是先用炮哥的代码把yolo的txt转换为xml;然后将所有的images和labels放入一个img的文件夹,然后用自己的代码划分训练集和验证集。
1、新建文件夹
在/data/下新建voc_data文件夹,在voc_data文件夹下新建Annotations,JPEGImages,YOLO
- Annotations:存放转换之后的xml标注
- JPEGImages:将解压后的images中的图片全部拷贝到此
- YOLO:将解压后的txt文件全部拷贝到次
2、将txt的标注转换为xml
在data目录下新建yolo_to_voc.py,注意main方法中的路径
from xml.dom.minidom import Document
import os
import cv2
# 参考链接:https://blog.csdn.net/didiaopao/article/details/120022845
# def makexml(txtPath, xmlPath, picPath): # txt所在文件夹路径,xml文件保存路径,图片所在文件夹路径
def makexml(picPath, txtPath, xmlPath): # txt所在文件夹路径,xml文件保存路径,图片所在文件夹路径
"""此函数用于将yolo格式txt标注文件转换为voc格式xml标注文件
在自己的标注图片文件夹下建三个子文件夹,分别命名为picture、txt、xml
"""
dic = {'0': "no-mask", # 创建字典用来对类型进行转换
'1': "mask", # 此处的字典要与自己的classes.txt文件中的类对应,且顺序要一致
}
files = os.listdir(txtPath)
for i, name in enumerate(files):
xmlBuilder = Document()
annotation = xmlBuilder.createElement("annotation") # 创建annotation标签
xmlBuilder.appendChild(annotation)
txtFile = open(txtPath + name)
print("文件:",txtPath + name)
txtList = txtFile.readlines()
img = cv2.imread(picPath + name[0:-4] + ".jpg")
Pheight, Pwidth, Pdepth = img.shape
folder = xmlBuilder.createElement("folder") # folder标签
foldercontent = xmlBuilder.createTextNode("driving_annotation_dataset")
folder.appendChild(foldercontent)
annotation.appendChild(folder) # folder标签结束
filename = xmlBuilder.createElement("filename") # filename标签
filenamecontent = xmlBuilder.createTextNode(name[0:-4] + ".jpg")
filename.appendChild(filenamecontent)
annotation.appendChild(filename) # filename标签结束
size = xmlBuilder.createElement("size") # size标签
width = xmlBuilder.createElement("width") # size子标签width
widthcontent = xmlBuilder.createTextNode(str(Pwidth))
width.appendChild(widthcontent)
size.appendChild(width) # size子标签width结束
height = xmlBuilder.createElement("height") # size子标签height
heightcontent = xmlBuilder.createTextNode(str(Pheight))
height.appendChild(heightcontent)
size.appendChild(height) # size子标签height结束
depth = xmlBuilder.createElement("depth") # size子标签depth
depthcontent = xmlBuilder.createTextNode(str(Pdepth))
depth.appendChild(depthcontent)
size.appendChild(depth) # size子标签depth结束
annotation.appendChild(size) # size标签结束
for j in txtList:
oneline = j.strip().split(" ")
object = xmlBuilder.createElement("object") # object 标签
picname = xmlBuilder.createElement("name") # name标签
namecontent = xmlBuilder.createTextNode(dic[oneline[0]])
picname.appendChild(namecontent)
object.appendChild(picname) # name标签结束
pose = xmlBuilder.createElement("pose") # pose标签
posecontent = xmlBuilder.createTextNode("Unspecified")
pose.appendChild(posecontent)
object.appendChild(pose) # pose标签结束
truncated = xmlBuilder.createElement("truncated") # truncated标签
truncatedContent = xmlBuilder.createTextNode("0")
truncated.appendChild(truncatedContent)
object.appendChild(truncated) # truncated标签结束
difficult = xmlBuilder.createElement("difficult") # difficult标签
difficultcontent = xmlBuilder.createTextNode("0")
difficult.appendChild(difficultcontent)
object.appendChild(difficult) # difficult标签结束
bndbox = xmlBuilder.createElement("bndbox") # bndbox标签
xmin = xmlBuilder.createElement("xmin") # xmin标签
mathData = int(((float(oneline[1])) * Pwidth + 1) - (float(oneline[3])) * 0.5 * Pwidth)
xminContent = xmlBuilder.createTextNode(str(mathData))
xmin.appendChild(xminContent)
bndbox.appendChild(xmin) # xmin标签结束
ymin = xmlBuilder.createElement("ymin") # ymin标签
mathData = int(((float(oneline[2])) * Pheight + 1) - (float(oneline[4])) * 0.5 * Pheight)
yminContent = xmlBuilder.createTextNode(str(mathData))
ymin.appendChild(yminContent)
bndbox.appendChild(ymin) # ymin标签结束
xmax = xmlBuilder.createElement("xmax") # xmax标签
mathData = int(((float(oneline[1])) * Pwidth + 1) + (float(oneline[3])) * 0.5 * Pwidth)
xmaxContent = xmlBuilder.createTextNode(str(mathData))
xmax.appendChild(xmaxContent)
bndbox.appendChild(xmax) # xmax标签结束
ymax = xmlBuilder.createElement("ymax") # ymax标签
mathData = int(((float(oneline[2])) * Pheight + 1) + (float(oneline[4])) * 0.5 * Pheight)
ymaxContent = xmlBuilder.createTextNode(str(mathData))
ymax.appendChild(ymaxContent)
bndbox.appendChild(ymax) # ymax标签结束
object.appendChild(bndbox) # bndbox标签结束
annotation.appendChild(object) # object标签结束
f = open(xmlPath + name[0:-4] + ".xml", 'w')
xmlBuilder.writexml(f, indent='\t', newl='\n', addindent='\t', encoding='utf-8')
f.close()
if __name__ == "__main__":
# picPath = "VOCdevkit/VOC2007/JPEGImages/" # 图片所在文件夹路径,后面的/一定要带上
# txtPath = "VOCdevkit/VOC2007/YOLO/" # txt所在文件夹路径,后面的/一定要带上
# xmlPath = "VOCdevkit/VOC2007/Annotations/" # xml文件保存路径,后面的/一定要带上
picPath = "voc_data/JPEGImages/" # 图片所在文件夹路径,后面的/一定要带上
txtPath = "voc_data/YOLO/" # txt所在文件夹路径,后面的/一定要带上
xmlPath = "voc_data/Annotations/" # xml文件保存路径,后面的/一定要带上
makexml(picPath, txtPath, xmlPath)
执行转换
python yolo_to_voc.py
转换之后在data/voc_data/Annotations中就都是xml了
注意数据集中有个labels是错误的,类型是none,需要删除否则程序会报错,可以在代码的21行加上print(“文件:”,txtPath + name),找到具体哪个文件有问题。
3、划分训练集和验证集
将voc_data/Annotations和JPEGImages都拷贝到data/img目录下
cp -rp data/voc_data/Annotations/* data/img/
cp -rp data/voc_data/JPEGImages/* data/img/
拷贝划分程序process-date文件夹到data目录下
注意修改create_all.py中的类别,然后执行
python create_all.py
本代码会自动划分训练集和验证集,并把错误的图片或者标注文件筛选出来
四、训练
我们使用yolov5l.pt的预训练模型来训练
1、准备mask.yml
在data目录下新建mask.yml。指定路径和类别
2、修改models/yolov5l.yaml
修改识别类型
3、新建train_mask.py
cp train.py train_mask.py
重点修改如下
epochs设置训练300轮
batch-size设置10,可以根据gpu的性能设置,默认是16
4、训练
python train_mask.py
5、生成的模型
五、推理验证
1、新建detect_mask.py
cp detect.py detect_mask.py
2、推理图片
python detect_mask.py --source data/test/
3、推理视频
下载行人视频
lux https://www.bilibili.com/video/BV1Q54y1L74D
推理
python detect_mask.py --source D:\ai\mask.mp4
六、转换瑞芯微
1、导出中间模型
修改models/yolo.py
修改注释,切记导出完之后改回去,否则推理,训练都会报错
导出
cp export.py export_mask.py
修改如下
python export_mask.py
会在best.pt目录下生成best.torchscript.pt
2、中间模型转换瑞芯微模型
rk工具路径:
/cnn/rknn/rknn-toolkit-master/examples/pytorch/yolov5
拷贝中间模型
将best.torchscript.pt拷贝到rk工具路径中
mv best.torchscript.pt /cnn/rknn/rknn-toolkit-master/examples/pytorch/yolov5/mask.torchscript.pt
新建convert_mask.py
代码如下,重点
- PT_MODEL = ‘mask.torchscript.pt’
- RKNN_MODEL = ‘mask.rknn’
import os
import numpy as np
import cv2
from rknn.api import RKNN
PT_MODEL = 'mask.torchscript.pt'
RKNN_MODEL = 'mask.rknn'
IMG_PATH = 'bus.jpg'
DATASET = './dataset.txt'
# QUANTIZE_ON = False
QUANTIZE_ON = True
BOX_THRESH = 0.5
NMS_THRESH = 0.6
IMG_SIZE = 640
CLASSES = ("person")
def sigmoid(x):
return 1 / (1 + np.exp(-x))
def xywh2xyxy(x):
# Convert [x, y, w, h] to [x1, y1, x2, y2]
y = np.copy(x)
y[:, 0] = x[:, 0] - x[:, 2] / 2 # top left x
y[:, 1] = x[:, 1] - x[:, 3] / 2 # top left y
y[:, 2] = x[:, 0] + x[:, 2] / 2 # bottom right x
y[:, 3] = x[:, 1] + x[:, 3] / 2 # bottom right y
return y
def process(input, mask, anchors):
anchors = [anchors[i] for i in mask]
grid_h, grid_w = map(int, input.shape[0:2])
box_confidence = sigmoid(input[..., 4])
box_confidence = np.expand_dims(box_confidence, axis=-1)
box_class_probs = sigmoid(input[..., 5:])
box_xy = sigmoid(input[..., :2])*2 - 0.5
col = np.tile(np.arange(0, grid_w), grid_w).reshape(-1, grid_w)
row = np.tile(np.arange(0, grid_h).reshape(-1, 1), grid_h)
col = col.reshape(grid_h, grid_w, 1, 1).repeat(3, axis=-2)
row = row.reshape(grid_h, grid_w, 1, 1).repeat(3, axis=-2)
grid = np.concatenate((col, row), axis=-1)
box_xy += grid
box_xy *= int(IMG_SIZE/grid_h)
box_wh = pow(sigmoid(input[..., 2:4])*2, 2)
box_wh = box_wh * anchors
box = np.concatenate((box_xy, box_wh), axis=-1)
return box, box_confidence, box_class_probs
def filter_boxes(boxes, box_confidences, box_class_probs):
"""Filter boxes with box threshold. It's a bit different with origin yolov5 post process!
# Arguments
boxes: ndarray, boxes of objects.
box_confidences: ndarray, confidences of objects.
box_class_probs: ndarray, class_probs of objects.
# Returns
boxes: ndarray, filtered boxes.
classes: ndarray, classes for boxes.
scores: ndarray, scores for boxes.
"""
box_classes = np.argmax(box_class_probs, axis=-1)
box_class_scores = np.max(box_class_probs, axis=-1)
pos = np.where(box_confidences[...,0] >= BOX_THRESH)
boxes = boxes[pos]
classes = box_classes[pos]
scores = box_class_scores[pos]
return boxes, classes, scores
def nms_boxes(boxes, scores):
"""Suppress non-maximal boxes.
# Arguments
boxes: ndarray, boxes of objects.
scores: ndarray, scores of objects.
# Returns
keep: ndarray, index of effective boxes.
"""
x = boxes[:, 0]
y = boxes[:, 1]
w = boxes[:, 2] - boxes[:, 0]
h = boxes[:, 3] - boxes[:, 1]
areas = w * h
order = scores.argsort()[::-1]
keep = []
while order.size > 0:
i = order[0]
keep.append(i)
xx1 = np.maximum(x[i], x[order[1:]])
yy1 = np.maximum(y[i], y[order[1:]])
xx2 = np.minimum(x[i] + w[i], x[order[1:]] + w[order[1:]])
yy2 = np.minimum(y[i] + h[i], y[order[1:]] + h[order[1:]])
w1 = np.maximum(0.0, xx2 - xx1 + 0.00001)
h1 = np.maximum(0.0, yy2 - yy1 + 0.00001)
inter = w1 * h1
ovr = inter / (areas[i] + areas[order[1:]] - inter)
inds = np.where(ovr <= NMS_THRESH)[0]
order = order[inds + 1]
keep = np.array(keep)
return keep
def yolov5_post_process(input_data):
masks = [[0, 1, 2], [3, 4, 5], [6, 7, 8]]
anchors = [[10, 13], [16, 30], [33, 23], [30, 61], [62, 45],
[59, 119], [116, 90], [156, 198], [373, 326]]
boxes, classes, scores = [], [], []
for input,mask in zip(input_data, masks):
b, c, s = process(input, mask, anchors)
b, c, s = filter_boxes(b, c, s)
boxes.append(b)
classes.append(c)
scores.append(s)
boxes = np.concatenate(boxes)
boxes = xywh2xyxy(boxes)
classes = np.concatenate(classes)
scores = np.concatenate(scores)
nboxes, nclasses, nscores = [], [], []
for c in set(classes):
inds = np.where(classes == c)
b = boxes[inds]
c = classes[inds]
s = scores[inds]
keep = nms_boxes(b, s)
nboxes.append(b[keep])
nclasses.append(c[keep])
nscores.append(s[keep])
if not nclasses and not nscores:
return None, None, None
boxes = np.concatenate(nboxes)
classes = np.concatenate(nclasses)
scores = np.concatenate(nscores)
return boxes, classes, scores
def draw(image, boxes, scores, classes):
"""Draw the boxes on the image.
# Argument:
image: original image.
boxes: ndarray, boxes of objects.
classes: ndarray, classes of objects.
scores: ndarray, scores of objects.
all_classes: all classes name.
"""
for box, score, cl in zip(boxes, scores, classes):
top, left, right, bottom = box
print('class: {}, score: {}'.format(CLASSES[cl], score))
print('box coordinate left,top,right,down: [{}, {}, {}, {}]'.format(top, left, right, bottom))
top = int(top)
left = int(left)
right = int(right)
bottom = int(bottom)
cv2.rectangle(image, (top, left), (right, bottom), (255, 0, 0), 2)
cv2.putText(image, '{0} {1:.2f}'.format(CLASSES[cl], score),
(top, left - 6),
cv2.FONT_HERSHEY_SIMPLEX,
0.6, (0, 0, 255), 2)
def letterbox(im, new_shape=(640, 640), color=(0, 0, 0)):
# Resize and pad image while meeting stride-multiple constraints
shape = im.shape[:2] # current shape [height, width]
if isinstance(new_shape, int):
new_shape = (new_shape, new_shape)
# Scale ratio (new / old)
r = min(new_shape[0] / shape[0], new_shape[1] / shape[1])
# Compute padding
ratio = r, r # width, height ratios
new_unpad = int(round(shape[1] * r)), int(round(shape[0] * r))
dw, dh = new_shape[1] - new_unpad[0], new_shape[0] - new_unpad[1] # wh padding
dw /= 2 # divide padding into 2 sides
dh /= 2
if shape[::-1] != new_unpad: # resize
im = cv2.resize(im, new_unpad, interpolation=cv2.INTER_LINEAR)
top, bottom = int(round(dh - 0.1)), int(round(dh + 0.1))
left, right = int(round(dw - 0.1)), int(round(dw + 0.1))
im = cv2.copyMakeBorder(im, top, bottom, left, right, cv2.BORDER_CONSTANT, value=color) # add border
return im, ratio, (dw, dh)
if __name__ == '__main__':
# Create RKNN object
rknn = RKNN(verbose=False)
if not os.path.exists(PT_MODEL):
print('model not exist')
exit(-1)
_force_builtin_perm = False
# pre-process config
print('--> Config model')
rknn.config(
reorder_channel='2 1 0',
mean_values=[[0, 0, 0]],
std_values=[[255, 255, 255]],
optimization_level=3,
# target_platform = 'rk1808',
target_platform='rv1126',
quantize_input_node= QUANTIZE_ON,
output_optimize=1,
force_builtin_perm=_force_builtin_perm)
print('done')
# Load ONNX model
print('--> Loading model')
ret = rknn.load_pytorch(model=PT_MODEL, input_size_list=[[3,640, 640]])
if ret != 0:
print('Load yolov5 failed!')
exit(ret)
print('done')
# Build model
print('--> Building model')
ret = rknn.build(do_quantization=QUANTIZE_ON, dataset=DATASET, pre_compile=True)
if ret != 0:
print('Build yolov5 failed!')
exit(ret)
print('done')
# Export RKNN model
print('--> Export RKNN model')
ret = rknn.export_rknn(RKNN_MODEL)
if ret != 0:
print('Export yolov5rknn failed!')
exit(ret)
print('done')
# # exit()
# # init runtime environment
# print('--> Init runtime environment')
# # ret = rknn.init_runtime()
# ret = rknn.init_runtime('rv1109', device_id='1109')
# # ret = rknn.init_runtime('rk1808', device_id='1808')
# if ret != 0:
# print('Init runtime environment failed')
# exit(ret)
# print('done')
# # Set inputs
# img = cv2.imread(IMG_PATH)
# img, ratio, (dw, dh) = letterbox(img, new_shape=(IMG_SIZE, IMG_SIZE))
# img = cv2.cvtColor(img, cv2.COLOR_BGR2RGB)
# # Inference
# print('--> Running model')
# outputs = rknn.inference(inputs=[img], inputs_pass_through=[0 if not _force_builtin_perm else 1])
# # post process
# input0_data = outputs[0]
# input1_data = outputs[1]
# input2_data = outputs[2]
# input0_data = input0_data.reshape([3,-1]+list(input0_data.shape[-2:]))
# input1_data = input1_data.reshape([3,-1]+list(input1_data.shape[-2:]))
# input2_data = input2_data.reshape([3,-1]+list(input2_data.shape[-2:]))
# input_data = list()
# input_data.append(np.transpose(input0_data, (2, 3, 0, 1)))
# input_data.append(np.transpose(input1_data, (2, 3, 0, 1)))
# input_data.append(np.transpose(input2_data, (2, 3, 0, 1)))
# boxes, classes, scores = yolov5_post_process(input_data)
# img_1 = cv2.cvtColor(img, cv2.COLOR_RGB2BGR)
# if boxes is not None:
# draw(img_1, boxes, scores, classes)
# cv2.imshow("post process result", img_1)
# cv2.waitKeyEx(0)
rknn.release()
3、执行转换
python convert_mask.py
则会在当前目录下生成mask.rknn
- 分享
- 举报
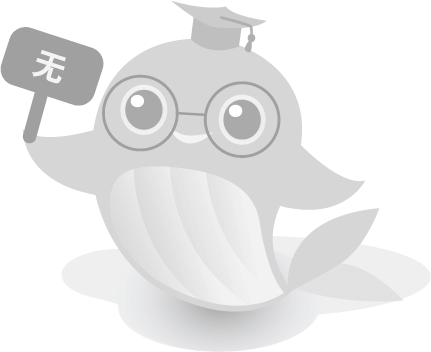
-
浏览量:210次2024-01-18 18:05:38
-
浏览量:423次2023-09-08 15:20:45
-
浏览量:260次2024-01-22 17:46:51
-
浏览量:348次2024-02-05 10:41:25
-
浏览量:431次2024-02-02 17:13:35
-
浏览量:152次2023-09-25 14:19:19
-
浏览量:213次2024-03-06 16:15:59
-
浏览量:471次2024-01-05 16:46:11
-
浏览量:6561次2021-01-08 02:45:30
-
浏览量:5875次2021-01-08 02:27:20
-
浏览量:229次2023-08-03 15:44:04
-
浏览量:254次2024-02-23 17:41:04
-
浏览量:143次2024-02-19 17:07:05
-
浏览量:346次2023-12-19 16:06:28
-
浏览量:1725次2022-10-09 10:38:23
-
浏览量:8810次2021-01-08 01:26:48
-
浏览量:5072次2021-01-08 03:12:37
-
浏览量:241次2024-02-19 15:26:47
-
浏览量:295次2023-12-27 15:46:55
-
广告/SPAM
-
恶意灌水
-
违规内容
-
文不对题
-
重复发帖
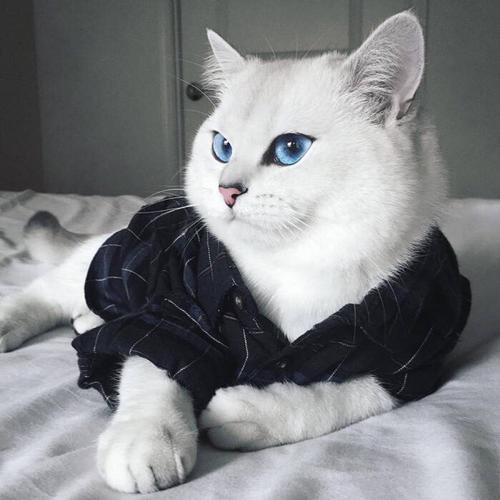
tomato






举报类型
- 内容涉黄/赌/毒
- 内容侵权/抄袭
- 政治相关
- 涉嫌广告
- 侮辱谩骂
- 其他
详细说明